Many of the common challenges in cosmetic ingredient and formulation optimization can be framed in terms of interactions on the molecular level:
- How can I reformulate using more sustainable ingredients without affecting performance?
- How do the ingredients in my formulation interact with its packaging?
- How does hair or skin respond to different ingredients during product application?
To answer these molecular-level questions, researchers need tools that simulate and predict molecular interactions – tools which enable them to connect experimental observations to their molecular origins, informing new perspectives from which to approach their research.
Accelerating Formulation Innovation with Digital Chemistry
Driven by the competitive need to innovate faster for the cosmetic and personal care industries, researchers have long applied Design of Experiments (DoE) in their product development and innovation programs. Often it is inefficient to test all possible combinations and proportions of ingredients in a formulation. Additionally, it’s expensive and time-consuming to synthesize new molecules or isolate ingredients from plant-based sources. Predictive models rooted in statistical analysis allow us to test just a subset of the possibilities and make educated guesses at the best next set of experiments to run, zeroing in on target properties.
Just like DoE’s revolutionized formulation design 100 years ago, digital chemistry is pushing formulations to new frontiers. Digital chemistry strategies can be viewed as the modern equivalent of traditional DoEs. The overarching goal of digital chemistry is to enable researchers to leverage their own data, alongside the principles of chemistry and physics, to design new molecules or formulations effectively and efficiently. Chemically-aware machine learning (ML) models have enhanced our ability to create models that can predict properties based on specific molecular characteristics of a system of interest. Detailed quantitative information about chemical structures, reactivity, and response to environmental factors can be encoded into models, expanding both their reliability and range of applicability.
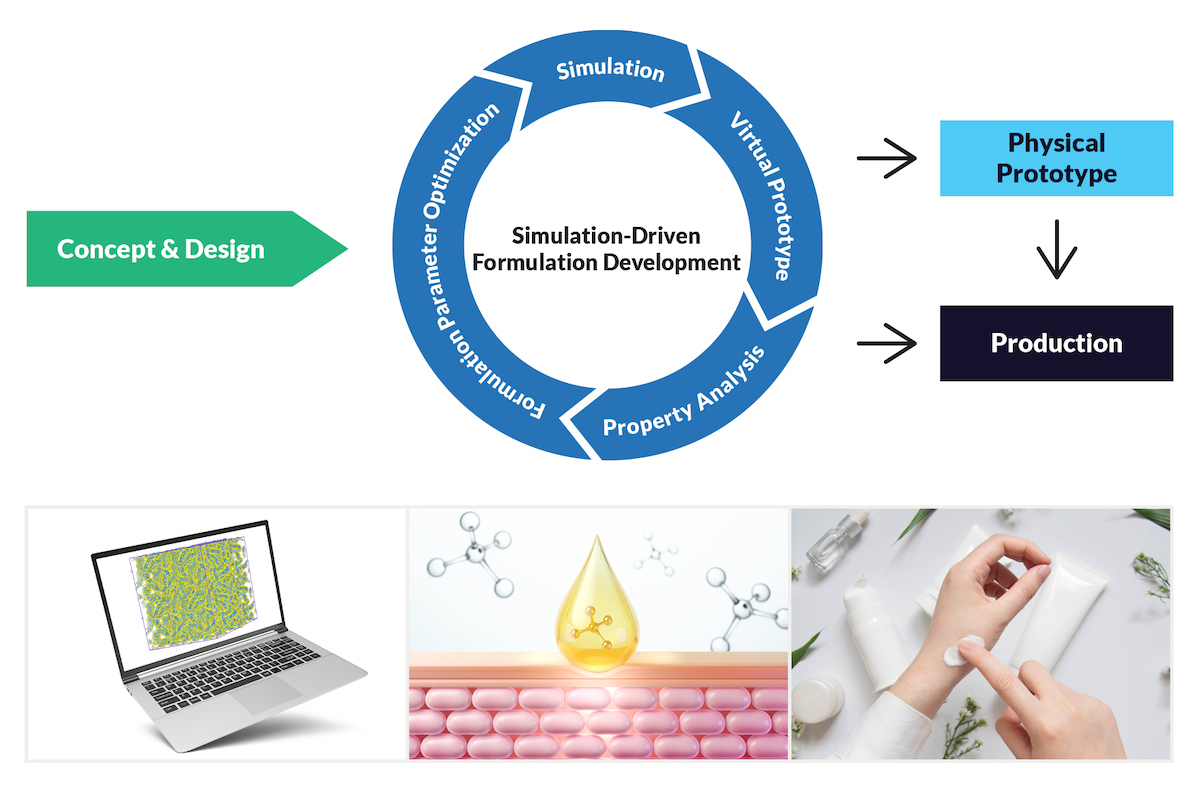
Figure 1. Simulation-driven formulation development enables faster innovation by screening and de-risking new ideas prior to experimental evaluation.
Physics-based molecular simulation can be a powerful addition to any R&D digitization strategy. Molecular simulations are computer-based tools that allow scientists to create 3D models of a system, starting with chemical structures, and then directly observe the behavior of its molecules with atomic resolution.
For example, surfactants mixed with oil and water will spontaneously assemble into a small vesicular aggregate. This same simulation experiment can be performed with variations on the types of oils, surfactants or both, and scientists can begin to establish systematic relationships between formula composition and aggregation behavior. Rather than run a dynamic light scattering experiment—a common experimental tool for characterization of surfactant solution behavior—for every new formulation idea, researchers can easily screen new ideas and then choose to devote experimental resources to only the most promising systems, thereby decreasing overall risk. Importantly, since the variations in molecules are not limited by the ingredients available through a chemical supplier, scientists have freedom to explore new ideas.
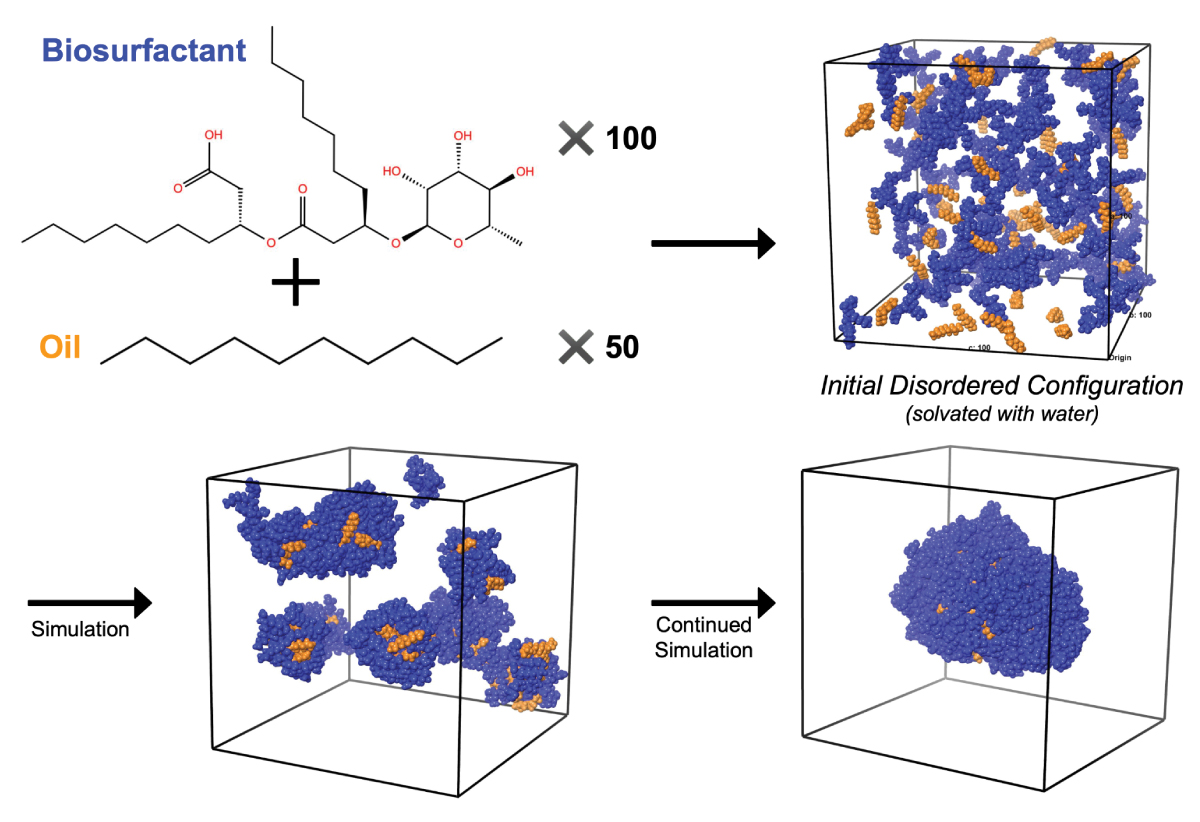
Figure 2. Biosurfactants mixed with oil and water (hidden from diagram) spontaneously form a small vesicular aggregate over the course of a simulation.
Active learning is an increasingly popular approach to experimentation, which combines both ML and molecular simulation. In a typical active learning application, batches of simulation experiments are run iteratively, incorporating new information into the ML model after each iteration. The systems tested during each iteration after initialization are chosen based on the predictions of the ML model. An active learning strategy towards formulation design is described below.
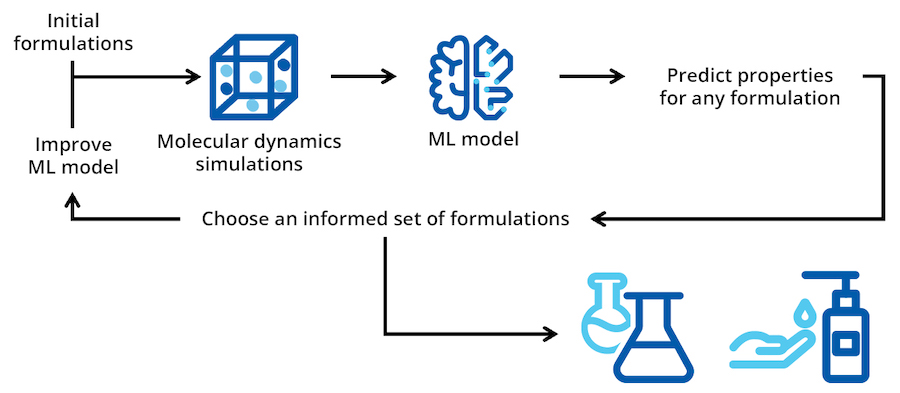
Figure 3. Active learning can be used to iteratively explore or narrow your design space by choosing batches of molecular simulations to run based on ML predictions. The most promising systems can then be tested in the lab.
While ML alone excels at interpolating data, molecular simulation enables extrapolation by supplementing missing data points. With molecular simulation, scientists can imagine and simulate a huge range of molecularly-sized systems from scratch. It’s easy to add new dimensions to an experimental design based purely on chemical intuition. Similar to DoEs, we can create an ML model based on a small subset of systems which helps us to navigate design space in the direction of ideal properties.
Leveraging Molecular Simulation for Sustainable Hair Formulations
Recently, Schrödinger collaborated with L’Oréal to understand the effect of replacing petroleum-derived polymers with sustainably-sourced polymers in hair care formulations. Simulations of molecular models of the surface of hair in contact with shampoo formulations showed polymer-dependent differences in surface interactions as well as bulk aggregation behavior. Measurements of friction under shear indicate stick-slip behavior that is dependent on polymer architecture. The methods established in this work will be applied towards high-throughput studies of more variations of the systems studied. For more details, please have a look at our joint publication.
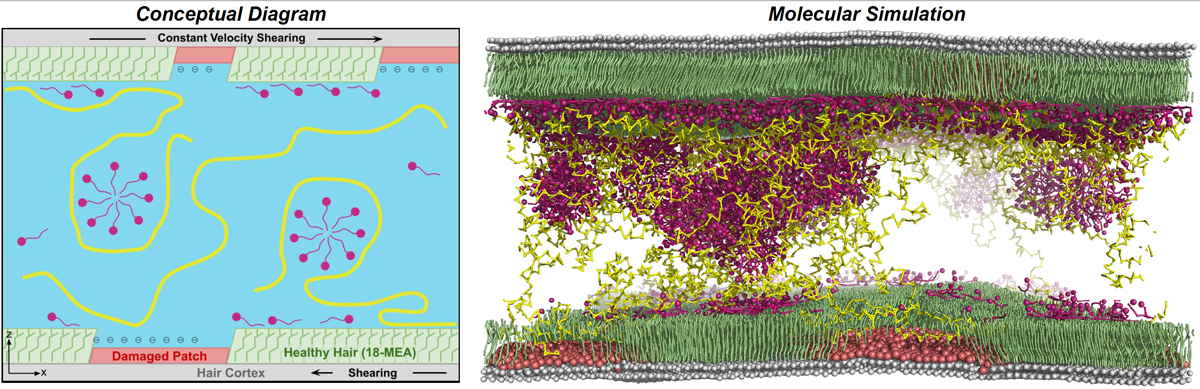
Figure 4. Beginning with an initial experimentally-inspired vision of the microscopic system, the molecular model consists of a bulk solution with polymers (yellow) and surfactants (pink) in contact with a partially damaged surface.
Integrating Simulations into Your R&D with Ease
Despite the significant value of molecular simulation to product development, there’s often a lingering misconception that the field is confined to academia or accessible only to domain experts. While this may have been true historically, the past decade has been accompanied by rapid advancement of both hardware and software solutions for simulation.
From a software perspective, it’s never been easier to get started running and extracting meaningful results from simulations. It’s no longer a requirement to juggle proficiency in coding and software development with in-depth knowledge of chemistry, quantum mechanics and statistical mechanics. Schrödinger’s mission is to provide software tools with easy-to-use graphical interfaces that place these powerful techniques into the hands of chemists and engineers with no prior simulation experience.
From a hardware perspective, cloud computing services continue to revolutionize the field by making high performance compute resources containing both CPU and graphics processing units (GPUs), colloquially termed “supercomputers,” accessible to anybody with an internet connection. In the past, industrial researchers depended on internally maintained computer clusters or public resources, oftentimes competing with other researchers’ access. The capital costs and setup required to follow this path are enough to delay or completely halt a foray into the world of molecular simulation. Cloud resources are flexible in implementation and enable simulation at any scale, subject to your own project requirements.
Even with these advances, simulation may still sound daunting. Fortunately, an abundance of education and training materials are available to help demystify the world of molecular simulation and enable users to make informed choices for their simulation conditions and for post-analysis. Many users find that simulations are easiest to grasp through practical application. Schrödinger offers hands-on courses and tutorials to guide users through relevant examples in consumer goods, polymers and more. Additionally, our applications scientists are available to help frame your specific research and development pressure points and interests in terms of tractable simulations, and a team of support scientists are here to ensure quick resolution of any other software-related issues.
Diving into molecular simulation may sound intimidating initially, but the recent strides in accessibility of the techniques promise a bright future. Surely, there are plenty of brilliant minds unaware of their aptitude for the field. If you find simulations intriguing, there has never been a better time to get started.
Learn more about Schrodinger’s education materials, visit our training website.
For more insights on molecular modeling across industry applications visit our Extrapolations website.